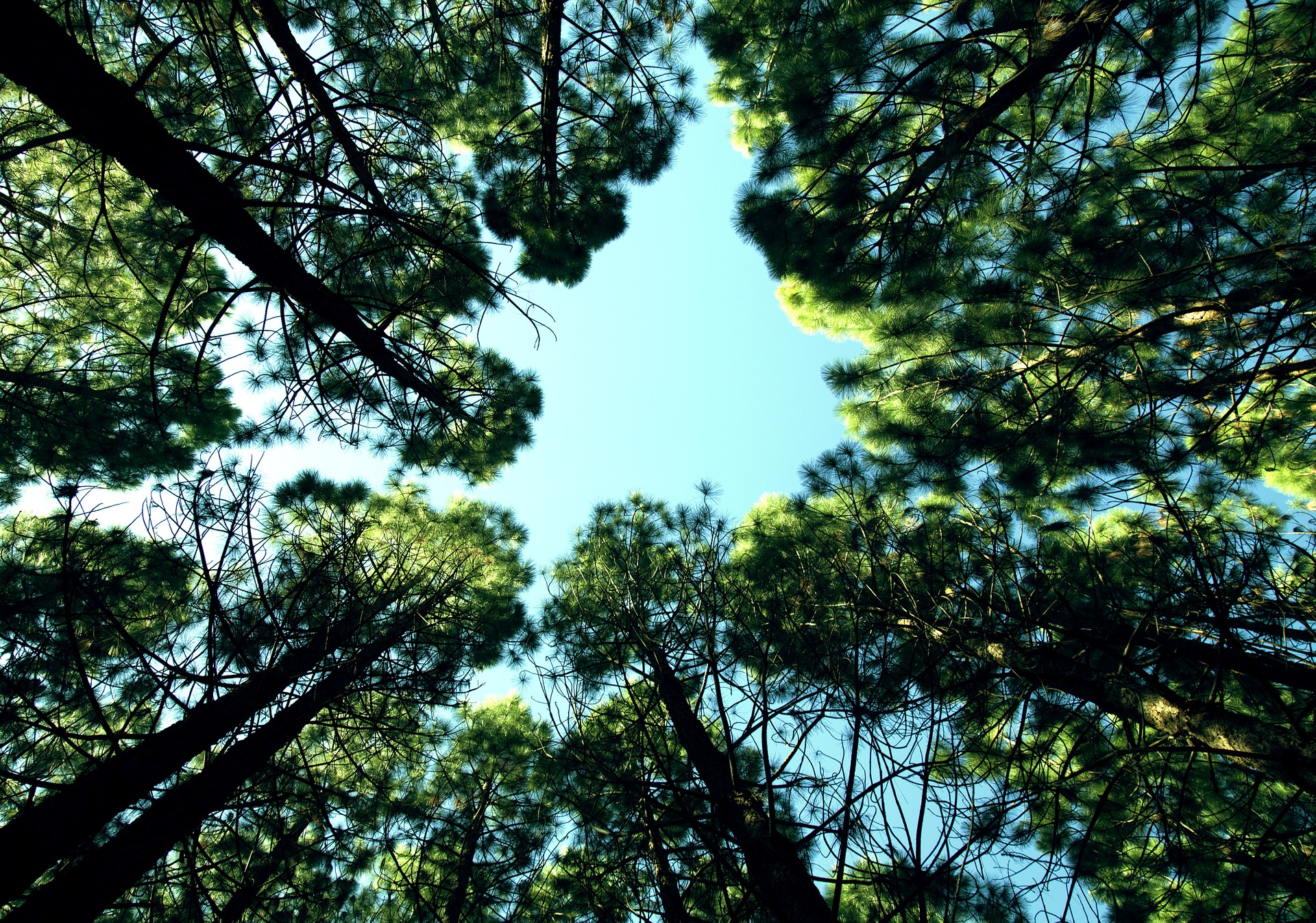
Over the last weeks, I have been in several group conversations where we had to agree on the relative priority of multiple factors. For instance, are we optimizing for the total number of users or are we optimizing for maximizing revenue per user? Should we prioritize fuel efficiency or is minimizing exhaust waste more important? Should we seek to get as much value from the initial sale of the system or should we aim to optimize the service and maintenance fees during the lifetime of the system? In all these situations, it rapidly became obvious that there were very different opinions in the room and that even individual participants were far from clear themselves.
Not being entirely clear on the relative priority of different relevant factors used to be fine as R&D was mostly driven by requirements provided by product management. And the product managers would have, sometimes extensive, discussions on the relative importance of different requirements and prioritize the backlog accordingly.
More recently, however, we’re increasingly moving towards outcome-driven and AI-driven development (as discussed in a blog post from earlier this year). Outcome-driven development uses techniques such as A/B/n testing to determine which alternative for achieving a certain outcome performs better. However, in that case you need to be crystal clear to define in quantitative terms what the desired outcome is. Similarly, AI-driven development typically uses large (often labeled) data-sets to train a machine- or deep-learning model to classify, predict, etc. new data based on a training data-set. However, again it is critically important to precisely define what constitutes success.
The point I am trying to make is that most companies that are new to data-driven R&D practices fail to conduct the exercise to clearly express quantitatively what they are optimizing for. There are several reasons why this is the case. It requires a significant amount of confrontation and transparency to call out team members who seem to be optimizing for other factors than what we agreed upon. Deciding on the relative priority of factors requires the organization to make hard choices. In my experience, when decision makers are asked to choose between alternative A and B, the typical response is “I want both”.
Failing to get clear on relative priorities and making real choices, however, results in several dysfunctions and inefficiencies in organizations. For example, in one company, one team worked on improving the security of the product and another team was looking to improve performance. The actions of both teams cancelled out each others efforts and the result was a lot of activity but no progress whatsoever.
Concluding, as we’re increasingly adopt data-driven practices such as outcome- and AI-driven development, we can no longer afford to be vague and imprecise on what we’re optimizing for. Instead, we need to engage in the hard discussions in the company to define clear relative priorities for the various factors that matter for the company and then engage in experiments to accomplish quantitatively validated improvements. In short, know what you’re optimizing for or pay the price!
To get more insights earlier, sign up for my mailing list at jan@janbosch.com or follow me on janbosch.com/blog, LinkedIn (linkedin.com/in/janbosch) or Twitter (@JanBosch).