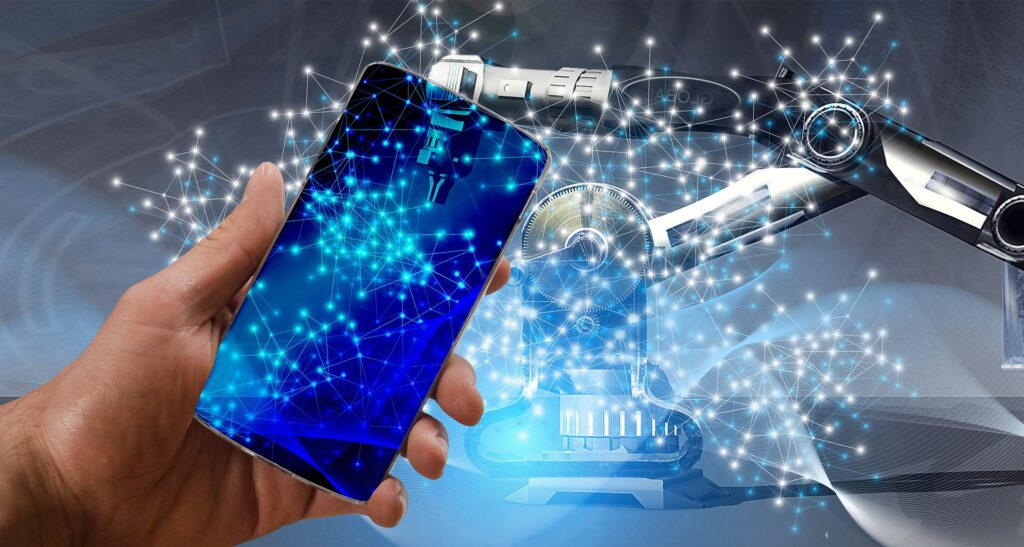
Thanks to fantastic amounts of investment and an ‘arms race’ between companies and between countries and regions, the progress in artificial intelligence has been phenomenal. Every day, a new model or new capability is announced somewhere on the globe. Although I could imagine an AI bubble bursting like the internet bubble did in 2000, the ‘peace dividend’ that AI technology brings to society and industry is still astounding.
As mentioned before, one of the patterns I’ve identified in the conversations I’ve had around the adoption of AI is that people find it much easier to identify opportunities for using AI in other people’s jobs than in their own. This is, in my experience, caused by several factors. Here, we’ll focus on the most prevalent ones.
The first factor is that many in senior leadership tend to have a very poor understanding of AI technology and capabilities. These senior leaders are typically put under pressure by their board to initiate AI projects to not miss the boat. The consequence tends to be that some techies are tasked with building a bunch of prototypes that look good in board meetings, but that fail to have any significant impact on the company as a whole.
The second factor, which exacerbates the first one, is that in many companies, there’s a significant gap between the business side and the technology side. This means not only that the business side doesn’t understand the technological capabilities of AI, but also that technology doesn’t understand the business strategy and the role AI could play in realizing it.
A third factor that comes up quite frequently is that many individuals in companies have outsized expectations of what can be done and focus on buzzwords. This leads to a focus on really disruptive efforts that, for a variety of reasons, are difficult to realize while failing to identify the low-hanging fruit that would provide immediate and significant benefit. Simple automation tasks are ignored and not considered “sexy” enough to be prioritized.
A fourth factor is a lack of ownership. I’ve met with companies where the CEO explicitly expected everyone to adopt AI. This leads to a situation where nobody owns the AI initiative and it gets stuck in a quagmire between business units, technology units and strategy units. Saying that everyone owns it is the same as saying nobody does.
As a fifth and final factor, many I talk to are underestimating the deployment of AI in existing workflows, processes and systems. The integration of an ML component or AI agent in a complex context not designed for incorporating the component or agent is a significant endeavor and tends to consume significantly more time and effort than the development of the AI part itself.
Of course, the general organizational resistance and the fear, uncertainty and doubt (FUD) that individuals tend to have about their own future in the company when AI can take over so many tasks are major concerns as well, but these will be discussed in a future post.
When we switch from a problem perspective to a solution orientation, the question obviously is how we address this. In the cases we studied where things worked well, three things were in place: ownership and authority, a structured framework for use case identification and a systematic deployment process.
First, the ownership of the AI initiative needs to be clearly assigned to an individual or team. Often, this is a central AI expert team, but such a model only works if two enablers are in place: the team, or at least its leader, needs to have a strong business orientation and understand the company’s business and strategy, and the team needs to have the authority to go into areas for which others are responsible and propose and implement changes. Of course, collaboration is preferred, but especially in highly decentralized organizations, there will be a strong tendency to keep central functions out by stonewalling or other techniques. The central AI team needs to have sufficient mandate to overcome such cultural tendencies.
Second, use cases need to flow through a clear process of identification, business impact assessment, technology assessment, prototyping and maturation. The goal is to determine whether an identified use case is relevant from a business perspective and feasible from a technology perspective. Of course, there also needs to be a process where use cases are prioritized based on business, technology capability, organizational willingness, risk, as well as other factors that may play a role.
One factor to consider here is the rapid evolution of AI technology. It may be perfectly reasonable to park a use case for a while when the exploratory phase makes it clear that the output isn’t of sufficient quality and revisit it a few months or a year later when technology has progressed further. Rather than taking a yes/no decision, the more reasonable viewpoint is to decide on now versus later.
Third, once a use case has been successfully prototyped and matured, the deployment needs to be careful and systematic. This usually requires more focus on organizational and human aspects than on technology aspects, as it often means that humans need to change their role from operational to supervisory, where they verify that the output created by the ML model or AI agent is indeed correct or of sufficient quality.
Many companies struggle with identifying and realizing AI use cases. This is caused by a variety of factors, including poor AI technology understanding by senior leadership, a gap between business and technology, unreasonable expectations, a lack of ownership and a lack of deployment capability. To address this, companies need to clearly assign ownership for AI initiatives and provide sufficient mandate to the AI team. In addition, they need a structured use case identification framework and, finally, a systematic deployment process where as much, if not more, attention is paid to organizational and human aspects as to AI technology. To end with a quote by Dave Waters: “Artificial intelligence is the future and the future is here!”
Want to read more like this? Sign up for my newsletter at jan@janbosch.com or follow me on janbosch.com/blog, LinkedIn (linkedin.com/in/janbosch) or X (@JanBosch).