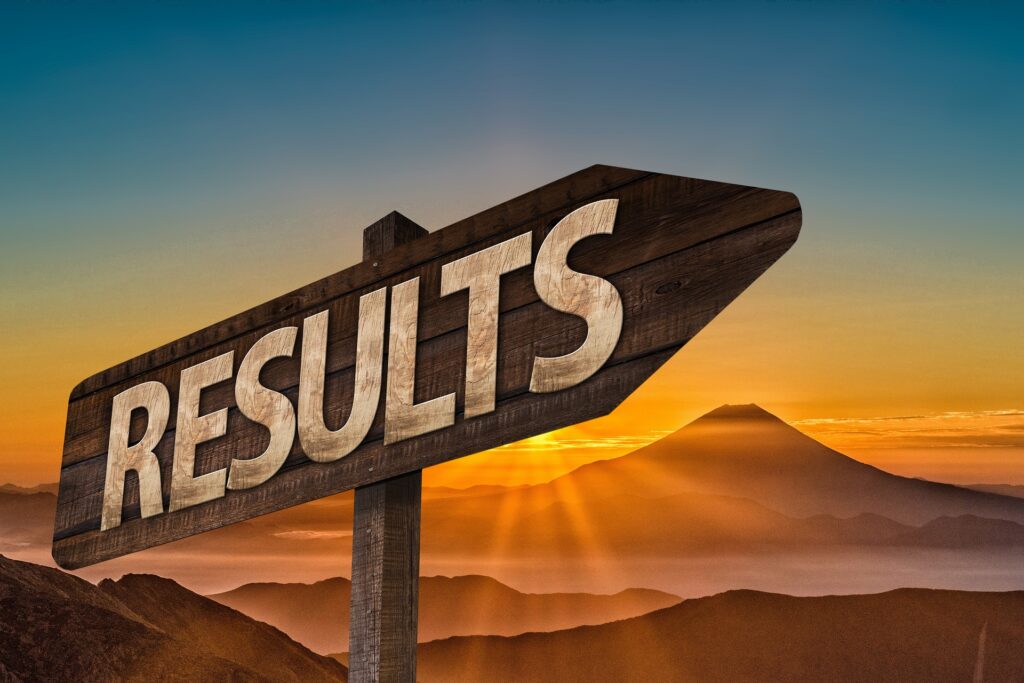
Humans are belief-driven storytelling machines who post-rationalize their, often irrational, behavior. This is at odds with our self-image of rational beings who make measured, balanced choices between well-reasoned alternatives. While this image may hold up in some contexts, research in psychology and elsewhere shows that unless there’s a non-negotiable yardstick holding us accountable, we easily resort to rationalizing unexpected outcomes after the fact as well as taking decisions beforehand on internally consistent but overall flawed lines of reasoning.
There are few more wonderful examples of this than all the news around the stock market. The explanations in the evening news of why shares moved up or down are often hilarious and mostly intended to give confused viewers and readers an explanation that resolves their cognitive dissonance while having little bearing on reality. Really funny are the people who are predicting developments in individual stocks or the market as a whole. All research shows that the accuracy of these so-called experts is about as good as rolling dice, ie completely random. The stock market, as well as many other systems involving humans, is a complex phenomenon for which we simply don’t have the models to make any prediction of reasonable accuracy. However, we feel the need to explain things and rather have incorrect explanations than admit that we simply don’t know.
Of course, as companies are made up of humans, the same patterns appear there. Various people inside the company predict the impact of actions, such as R&D investments. Especially in the planning of product development efforts, individuals argue for getting certain functionalities prioritized, claiming particular beneficial outcomes. These outcomes can be very concrete, eg “We’ll close the sale to this customer if we offer the requested functionality,” but they often are much less specific, eg “This will increase customer loyalty.” In practice, we seldom look back to evaluate the accuracy of these predictions. Instead, we’ve already moved on and are working on the next features to push for development while promising the most amazing benefits.
The best way I know to get around this problem is to define quantitative outcomes that we’re looking to accomplish with our investment in R&D. In SaaS companies, especially in e-commerce, this is often an easy problem: the only thing that matters and that we measure is conversion, ie the percentage of people coming to our site who actually buy something or otherwise do something beneficial. In companies where the offering includes mechanical, electronic and software parts, things often become more difficult. For instance, when asking a company providing commercial vehicles what the set of KPIs is that it’s optimizing for, the list easily gets very long. Of course, there’s uptime (or rather, unscheduled downtime), fuel efficiency, tire usage, driver comfort, gearbox effectiveness, average speed in hilly environments, and so on.
My experience is that most companies fall into the “worthwhile many versus vital few” trap: it’s not that these KPIs aren’t worthwhile to pursue, they are; it’s simply that there are so many of them that you can do anything and come up with a story on how it supports a relevant KPI. In addition, it provides no guidance on the relative priority of these factors. How much fuel efficiency do we need to achieve to allow for a 1 percent lowering of the vehicle’s uptime? In practice, many companies either have no explicitly stated KPIs or they have so many that it doesn’t offer a clear focus.
The D in Radical is concerned with data: we need to use data for our decision-making and prioritization. However, before we can do that, we first need to agree and decide on the vital few KPIs we’re pursuing as a business. And once we’ve done that, we can measure the impact of each activity as either impacting some of the vital KPIs positively without jeopardizing others or having no or negative impact.
To achieve this, we need to accomplish three milestones: define a value model, quantify each initiative and act on outcomes. The first step, as discussed, is to agree on the few vital KPIs that the company as a whole is pursuing. Although this may seem trivial in theory, getting everyone to agree on this proves to be surprisingly difficult in practice. However, to know that you’re making progress requires that you know where you’re trying to get to.
Second, every initiative, R&D or otherwise, should in some way quantitatively specify the expected impact on one or more of the few vital KPIs. This includes the primary KPIs that are intended to be affected as well as guardrail metrics representing aspects of the offering that can’t be affected negatively or aren’t allowed to sink below a certain threshold.
Third, we need to use the predictions and the actual outcomes as valuable input that allows us to learn and improve our ability over time. However, every initiative that doesn’t deliver sufficient impact should be stopped with the effects, such as code, removed from the system as soon as possible. Functionality that lives in the system but isn’t used only complicates the system, increases maintenance cost and may easily cause complicated quality issues.
Most companies don’t quantitatively specify the intended value delivery of their products. As a result, we fall back on our storytelling capabilities causing every initiative to, qualitatively, contribute in some way, shape or form to some worthwhile aspect of the system. We have no prioritization and we can’t measure the impact of our efforts. Instead, we need to quantitatively define the few, vital KPIs we pursue, express the intended impact of each R&D initiative in terms of these few KPIs and then measure the actual impact and compare it to the prediction. As Elbert Hubbard said: “It doesn’t take much strength to do things, but it requires a great deal of strength to decide what to do.”
Want to read more like this? Sign up for my newsletter at jan@janbosch.com or follow me on janbosch.com/blog, LinkedIn (linkedin.com/in/janbosch) or Twitter (@JanBosch).