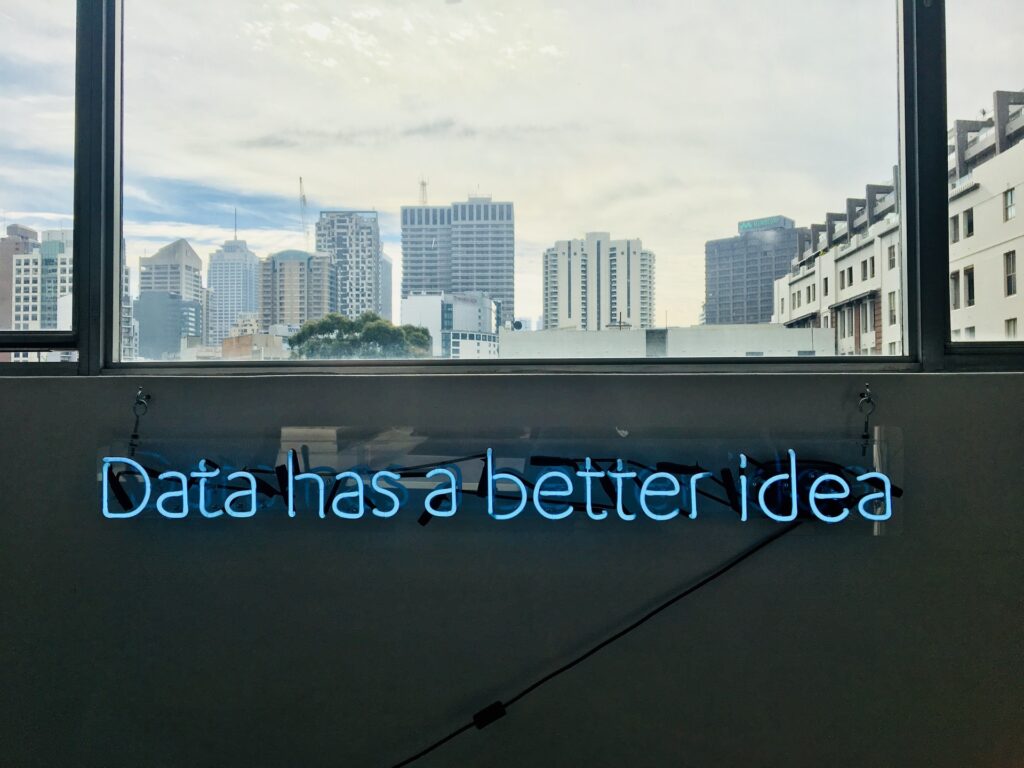
In this series, we have, time and again, stressed the importance of using data. We presented a variety of arguments to justify our focus on data, but they all center around the notion that, as humans, we’re highly able to create stories to explain why things are happening that, when more carefully inspected, turn out to be completely unfounded.
In addition, as we all collect experiences as we operate in our industry and work for our company, we increasingly use these experiences as a basis for our decision-making. The more we use them, the faster we can make decisions and the better we can explain why we advocate for a specific decision. However, in rapidly changing areas of expertise, for instance due to digitalization, the experiences we have are rapidly becoming invalid and we need to continuously critically investigate whether what we believe to be true indeed still is the case.
Few techniques are more effective in validating our beliefs than the use of data. We distinguish between qualitative data, like comments on our website or quotes from interviews with customers, and quantitative data, like results from surveys and A/B testing. Most often, however, people refer to quantitative data as the mechanism for making data-driven decisions.
The challenge is that working with quantitative data isn’t entirely trivial. Among data scientists, it’s well-known that by using the right statistical technique and carefully selecting data from a larger set, you can basically prove anything. As the saying goes, we have lies, big lies and statistics.
In addition, many moons ago, William Edwards Deming insisted that everyone should bring data. In an age where little quantitative data was available, the insistence on quantitative, statistically validated data was entirely understandable and the right focus. With the emergence of the big data era, however, we’ve entered a situation where the amount of available quantitative data is phenomenal and we’ve reached the other end of the spectrum: we have so much quantitative data that it becomes almost impossible to determine which data to use and for what purposes.
In our work with a variety of companies on data-driven practices, we’ve seen companies go through three phases. First, the company operates on traditional opinions, experiences and selected, qualitative customer input. In this phase, storytelling is an important part of the process and decisions are made based on the loudest customer, the stakeholder with the best rhetoric or the beliefs of the product manager.
When more data is being collected from the field and some of the decisions made earlier prove less than optimal, the second phase is entered. Here, everything the company seeks to do needs to be based on quantitative data. More and more data is collected, often as part of a “just in case” mindset: gather as much data as feasible and we’ll figure out ways to use it later. During this phase, two problems pop up: how to store, process and use all this data coming back from the field and, as the data savviness improves, the semantics of the data become increasingly unclear.
A typical example of this pattern is when the company starts to use A/B testing. When running multiple A/B tests at a time, statistically determining the impact of each experiment becomes increasingly challenging. The interaction problem between different A/B tests is a well-studied problem from an academic and research perspective and clear solution approaches, such as customer base segmentation and multi-factor analysis, are available. However, in practice, it’s surprisingly hard to avoid the challenges and interpret actual data to draw valid conclusions.
When the organization matures and becomes aware of the limitations of data, it enters the third phase. Here, qualitative and quantitative data are combined to draw conclusions that are both mathematically solid and have a clear qualitative meaning in the context of the company. When the company reaches this level, we see several behaviors occurring, including a proactive understanding of the why behind data, a healthy skepticism of conclusions drawn from data and a continuous discussion around the KPIs the company is optimizing for, ie value modeling.
First, whenever interesting and surprising insights are found in the data, more mature product managers seek to qualitatively understand the underlying explanation behind the data. This often requires collecting qualitative data from customers and other stakeholders, which may involve traditional techniques such as ethnographic studies, customer interviews and surveys with open questions.
Second, as it’s so easy to intentionally or unintentionally draw incorrect conclusions from data, there’s a healthy skepticism in mature organizations toward data-driven findings. In medicine, the rule is that for any new scientific truth to be accepted by the community, the same outcome has to have been achieved in at least three different studies by completely unrelated researchers. In mature, data-driven companies, the same principle is often applied: exceptional results require exceptional evidence.
Third, data-driven companies use data at all levels in the organization and the starting point often is a quantitative definition of the desired business strategy outcomes. Rather than a vague, qualitative “hand-waving” business strategy, these companies define clear, quantitative outcomes as well as the relative priorities of these outcomes in case initiatives affect multiple KPIs. In our research, we’ve used the term “value modeling” to refer to these activities. Ideally, there’s a clear, quantitative link between the business strategy and goals and the activities of individual teams in R&D and elsewhere in the organization.
Interestingly, even in traditional companies, finance and sales tend to be highly data-driven. The goal is to adopt data-driven practices in all other functions as well, including R&D. Done well, this can increase the effectiveness of R&D significantly, ie at least double it. Of course, it’s not necessarily trivial to achieve this, but considering the atrocious effectiveness of R&D in most companies, I remain flabbergasted at the fact that most companies stay with storytelling, qualitative approaches to prioritizing work in R&D.
Adopting data-driven practices is of critical importance to digital product management, but it’s not without challenges. It’s very easy to, basically, prove anything you want by selecting a suitable slice of the available data and applying a suitable statistical technique to it. Instead, we need to combine quantitative and qualitative data to ensure that we hold a set of beliefs about the product, the customers and the market that’s validated by quantitative data. For this, we need to constantly seek to understand the ‘why’ behind data, maintain a healthy skepticism toward conclusions based on data and ensure that we know what we’re optimizing for using value modeling. As William Turner so beautifully said: “You may have heard that the world is made up of atoms and molecules, but it really is made up of stories. When you sit with an individual who has been here, you can give quantitative data a qualitative overlay.”
Want to read more like this? Sign up for my newsletter at jan@janbosch.com or follow me on janbosch.com/blog, LinkedIn (linkedin.com/in/janbosch), Medium or Twitter (@JanBosch).