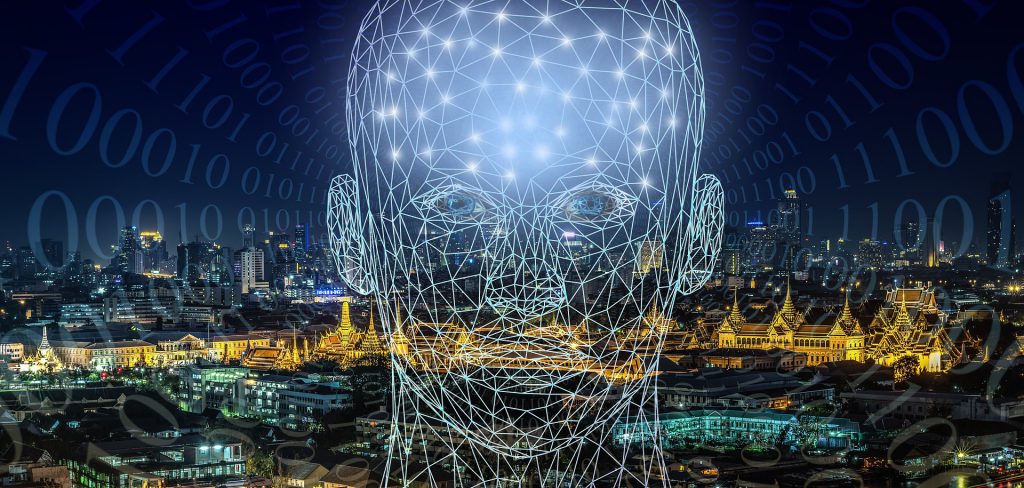
After two AI winters, artificial intelligence is now as hot as any topic can become. Over the last decade, entire research groups have been bought up by the large tech companies and the investment in all things AI has been phenomenal. The alignment of three major forces, ie the availability of data thanks to the big-data era, the GPU compute infrastructure thanks to the computer gaming industry and several breakthroughs in models and algorithms, has allowed for AI to become an increasingly critical component in many businesses.
ChatGPT, GPT4 and other large language models (LLMs) are fundamentally changing how we can interact with machines in the context of text as well as coding. Together with many others, I expect the productivity of software developers to go up 10x with the use of this technology. The same is true for other forms of generative AI, such as image generation, audio generation, video generation, and so on.
The challenge I see is that AI has led to quite a fundamental bifurcation in society. One group believes it’s akin to the second coming of Christ whereas another group thinks we’re on the way to Skynet and the extermination of humanity. Although there are some strong thinkers on both sides, many have very little clue of what they’re talking about and use the concept completely inaccurately.
There are many ways to provide some structure to the overall notion of AI, but one way to think about it is to break it into three main approaches: classical inference, generative AI and reinforcement learning. Classical inference is concerned with training a machine learning model with a data set for classification, prediction or recommendations. From a conceptual perspective, the model is a statistical model that has been trained by the training data and validated with a second data set.
Generative AI is concerned with machine learning models that generate text, images, videos, music, product designs, logos or whatever based on a prompt. These models tend to be extremely large and trained on vast amounts of data, but at its core, a generative AI model for text is a statistical model to generate the next word in a piece of text based on what other words were there already. The results are amazing, but in my view, we’re still in the “narrow AI” context rather than the artificial general intelligence (AGI) that many of the critics of AI are concerned about.
Reinforcement learning is concerned with systems experimenting with their own behavior to optimize their performance. It’s concerned with a state space, an action space and a reward function that’s learned over time. This area of AI is where I’m very excited about and where one of our PhD students is actively conducting research.
One of my big frustrations has been that many software-intensive systems have been static and have failed to evolve even if data shows their performance to be subpar. Of course, with the introduction of DevOps, we’ve reached a state where systems are becoming better over time, but the progress is often slow and applies equally to all products in the field. I want my system to learn about my behavior and adjust itself to how I want it to perform.
My favorite example is the adaptive cruise control in my car. It doesn’t work very well, in my opinion, so over the year or so that I’ve owned the car, I’ve learned how to interact with it so that the car behaves as I want it to. With reinforcement learning, we can get to a situation where the system learns over time based on the rewards you give it and you get your unique behavioral preference for your product.
My concern with the use of the term “AI” in the general public is threefold. First, many don’t really know what they mean with it, how it applies and in what way it is or isn’t relevant. This leads to very confused and misinformed discussions in society and the media.
Second, the only response governments, especially the EU, have seems to be regulation. Rather than using technology, the lawmakers seem akin to people with hammers: all they look for is nails. For instance, we can already use AI to determine if text was generated by an LLM or a human.
In addition, we’re looking to institute laws for characteristics that we as humans are not at all agreeing on. It’s easy to use terms like bias or fairness, but if we’re honest, it must be clear to everyone that there’s no consensus at all on what they mean in specific contexts. That leads to high degrees of uncertainty in companies looking to employ AI, which then causes many leaders to err on the side of caution. Especially in Europe, this results in adopting new technologies much later than other parts of the world, causing us to lose our competitive edge in yet another area.
Third, in the financial industry, one of the sayings is that the most dangerous words in investing are “this time it’s different.” That’s how bubbles are started and how many people lose fabulous amounts of money. AI is treated in this way whereas, in my view, it simply is another major technology shift that humanity has experienced before. Starting with the adoption of agriculture 12,000 years ago, followed by the industrial revolution in the 18th and 19th centuries, the adoption of computers in the 20th century and now the emergence of powerful AI solutions in the 21st century, humankind has always lived in times of change. Every one of these revolutions brings major shifts to society, but I think we can agree that as a species, humans have benefited tremendously from each of these and I have no reason to believe that this time it will be any different.
Finally, many are looking to predict what might go wrong with AI and then try to preempt the negative effects by regulation, protests, public opinion pieces and the like. The problem is that humans are terrible at prediction. One of my favorite examples is the horse manure crisis in New York in the late 1800s. As the city was growing rapidly, also the number of horses increased and the amount of manure produced by them was becoming a problem. There was a prediction that in fifty years, New York would be covered with more than two meters of horse shit based on the data. Of course, the automobile was introduced and the problem disappeared.
Humans are great at solving problems once these have occurred but terrible at predicting them from the outset. The world isn’t linear but rather a complex system where the effect of actions is very hard to foretell but often easy, or at least much easier, to address once challenges materialize. So, let’s stop trying to regulate and strangle innovation in AI and instead monitor whether things that we fear actually materialize and then, if they do, use technology instead of regulation to address them. As Rodney Brooks said: “AI is a tool, not a threat.”
Want to read more like this? Sign up for my newsletter at jan@janbosch.com or follow me on janbosch.com/blog, LinkedIn (linkedin.com/in/janbosch), Medium or Twitter (@JanBosch).