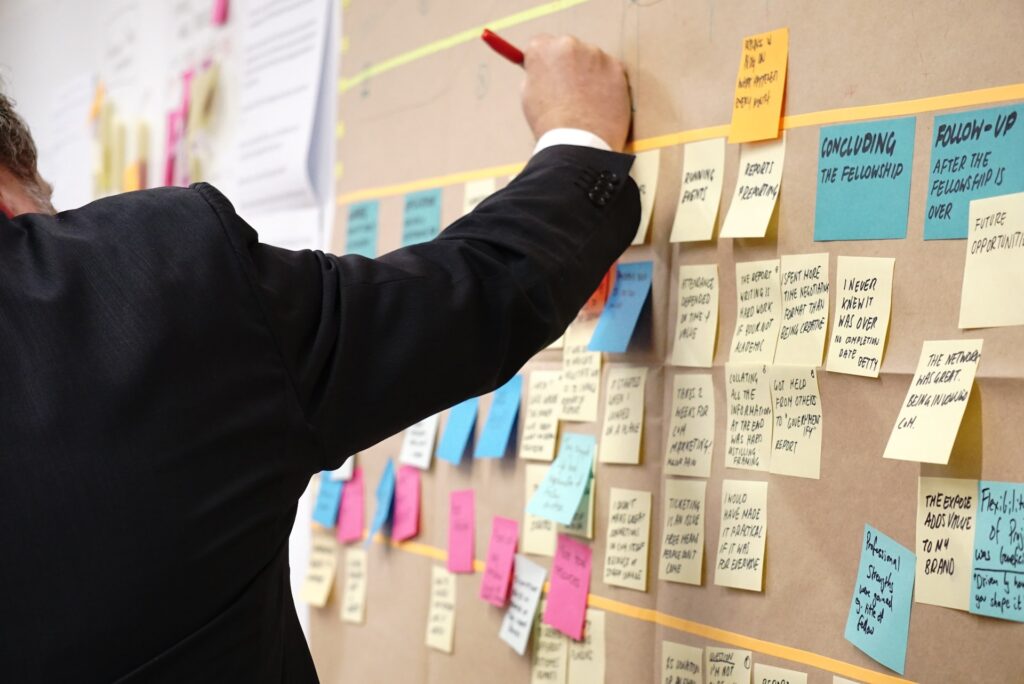
As human beings, we all have an innate need for security and safety. Much of the design of modern society is driven by this. We lock up criminals, especially violent ones, punish reckless behavior that might hurt others, design our environment to minimize our risk of getting hurt and enforce rules and regulations on products to ensure that these are safe.
In companies, we see the same behavior albeit expressed differently. In general, companies are organized into functions or departments. Each has its responsibility in the end-to-end value delivery process to customers. We have boundary objects on the interface between these departments such as customer orders, requirements specifications and budget allocations.
People don’t try to do the job of another department as that would intrude on their territory. Also, we don’t criticize the input we get through these boundary objects, at least not publicly, as it would denigrate the perceived competence of those providing the input. We don’t do these things as it would be very easy for others in the company to do the same to us, leading to a vicious cycle that doesn’t end well for anyone involved.
When the input we get from others doesn’t answer all the questions we might have, we can of course go and ask them, but in practice, that’s time consuming and tends to reflect badly on us if we do it too often. So, instead, we do what every engineer does: we fill in the blanks based on our assumptions about what should have been there. advertorial
Many in R&D are focused on the specification of a new product or a new feature in an existing product. The assumption is that it’s the job of product management to interact with the market and customers and to distill these insights into a specification that’s the optimal content. Reality shows that in practice, this is incorrect at multiple levels. First, it’s based on a generalization of the verbal input received by product managers. Second, it’s based on what customers say rather than on what they do.
The conclusion is that product management very often guesses the priority of the highest-impact activities and initiatives. Similar to how engineers often make design decisions based on their best understanding and experience, rather than driven by data. This isn’t because people are stupid or inexperienced, but simply because certain things are unknowable. There’s simply no way to predict the impact of new functionality or features on customer behavior and the market at large. The only way to find out is to experiment.
In my experience, three principles help address this fallacy: treating requirements as hypotheses, quantification of expected outcomes and continuous deployment. First, many in R&D tend to treat requirements as cast in stone and written in blood: immutable, unquestionable and meeting the requirements is the only thing that counts. In practice, a requirement is nothing but a hypothesis about what might add value to customers. Treating a requirement as a hypothesis and then finding smart, cost-effective ways to validate the hypothesis by iteratively adding confidence is a much more productive approach.
Second, in some of the companies I work with, R&D teams have now learned to immediately ask product managers who come with a feature request what the quantitative, measurable outcome of that feature is expected to be – how system behavior or customer behavior is expected to change in response to the feature. This shifts the nature of the conversation from the requirement to the intended outcome, which then allows for a much more free and open discussion around how to best realize that outcome.
Third, we’re looking to minimize investment in new functionality until it has proven itself. The best way to do this is by iteratively building slices of the functionality, releasing each slice and measuring the effect. This of course requires the continuous deployment of software to customers, but also instrumentation so that we can baseline and measure the effect of each slice. In an earlier post, I discussed the Hypex model in more detail, which is a good way to realize this.
Many in R&D tend to use the requirements specification as an absolute, rather than as a list of hypotheses concerning functionality that might add value to customers. This is because the specification is typically used as the boundary object between product management and development, and these boundary objects are typically not questioned. This leads to low effectiveness of R&D as research shows that many features aren’t used in practice. Instead, we need to treat each requirement as a hypothesis, quantify the intended effect or outcome and then iteratively develop the requirement to gather evidence that the hypothesis is valid. And, of course, we should kill the development of a feature when the data shows that there’s no effect. To quote Peter Drucker, efficiency is doing things right, effectiveness is doing the right things. R&D has traditionally focused on efficiency, but in a digital world, it needs to focus on effectiveness instead.
Like what you read? Sign up for my newsletter at jan@janbosch.com or follow me on janbosch.com/blog, LinkedIn (linkedin.com/in/janbosch), Medium or Twitter (@JanBosch).