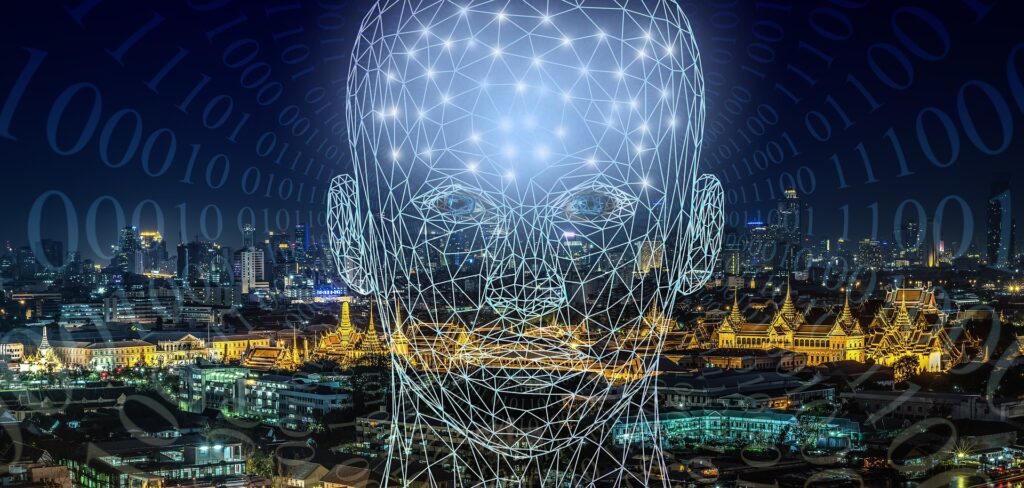
Every few weeks, it seems, we are treated to another major breakthrough innovation in artificial intelligence. Whether it is Deep Mind’s system beating the world champion Go player, GPT-3 dazzling us with Turing test breaking abilities or DALLE-2 generating images that are simply stunning, it seems like we are on the cusp of general artificial intelligence.
In reality, my view is that we are much less advanced than what these news making innovations seem to suggest, and for the typical company that I work with, many of the machine learning and deep learning (ML/DL) models are still living mostly in prototypes and experimental setups.
For companies to be successful in their digital transformation, it is not sufficient to use more software and collect more data. We also need to use this capability to collect data for more than traditional data analytics. The data provides an excellent basis for training ML/DL models as well and if we do not exploit this opportunity, others will and out-innovate us.
For all the hype around artificial intelligence, I still see quite a bit of hesitation to go beyond the prototypes into production. This is the case for, at least, four main reasons. First, putting a significant part of your offering’s functionality in the hands of a model that you don’t really understand, can’t inspect nor explain to customers is a hard thing to accept for anyone with a technical background. As a consequence, it is easy to decide to ask for more time, more evidence, more experimentation, etc. before you are willing to incorporate the model into a real offering.
Second, one of the key challenges of ML/DL models is their statistical nature. Whether you focus on accuracy, F1 scores or any other metric, the fact of the matter is that no model reaches 100%. This means that there will be cases, where the system simply acts incorrectly. Even if accuracy is 99.9%, it still means that, on average, once every 1000 instances, things go wrong. For companies that have thousands or millions of products out in the field, this leads to significant numbers of failures. Even if the model on average does much better than the algorithmically programmed solution, the uncertainty associated with the consequence of failures leads to a lack of deployment of these models.
One striking example for me is autonomous vehicles. The statistics show that these vehicles are much, much safer than human drivers, but of course still make mistakes with potentially lethal consequences. The mistakes by these ML/DL models, however, are experienced as much worse as mistakes by human drivers. Despite the many videos of autonomous functions in cars avoiding accidents where their human driver missed the danger, humanity apparently rather has tens of thousands of people per year die in traffic accidents than accept a much lower number of deaths due to autonomous vehicles.
The third reason why companies are slow in deploying AI solutions is the associated work that comes with it. Most ML/DL solutions simply are data hungry and perform better with increasing amounts of data. Collecting, cleaning, labelling and storing vast amounts of data in continuous data streams is a major investment and very labor intensive. This goes counter the general public’s view of throwing a ML/DL model at a bunch of Atari computer games and the system learning by itself. Most AI approaches are using supervised learning which typically requires the large data sets.
Fourth, some problems that may seem easy in concept prove to be surprisingly hard to get right in practice. Similar to the infamous Clippy by Microsoft in the 1990s, it may prove to be very challenging to provide solutions that provide the right predictions, classifications or recommendations and once system performance falls below a certain threshold, users are simply disgusted with the system and refuse to use the “intelligent” functions. Combined with the lack of AI expertise in most companies, many obvious use cases prove to simply be elusive with current knowledge, architecture and approaches.
Although the above reasons, as well as many others, may seem convincing to delay the adoption of AI, my point is the opposite: we do this because it is hard. The benefit of successfully deploy models that solve real problems that earlier were unsolved is significant and even if investing in AI is more risky than a run-of-the-mill innovation project, the fact is that AI represents an amazing general technology that affects all industries. That means, investing in continuous deployment, data pipelines to collect the data streams, etc. with the intent of also building the capabilities to successfully deploy ML/DL models over time.
Especially for embedded systems companies, however, there is one additional approach that often is ignored. Most AI these days is based on offline training using centralized data. However, embedded systems companies have thousands if not millions of systems out in the field that have, at different times, some excess computing capacity. This allows for federated learning approaches where you can have systems train their own models and exchange these with each other in order to continuously improve performance without having to bring all data to a central location. In addition, for less safety-critical use cases, we can use reinforcement learning to have systems experiment while in operation and share the findings with each other using federated approaches.
The holy grail, at least to me, is to have systems that are continuously improving their performance not just through continuous deployment of new software but also because these systems experiment with their own behavior and learn, over time, how to optimally operate in each specific situation and context. Once you have adopted A/B testing, the topic of the previous post, it is not such a large jump to identify areas of functionality where you allow the system to experiment autonomously.
Concluding, for all the hype around AI, many companies are slow in deploying ML/DL models in production. This may be because of the lack of explainability, the statistical nature of ML/DL models, the large amount of work setting up everything data and training related or the challenge of modeling the use case in such a way that we have acceptable performance. Still, AI is a general and transformational technology that offers many advantages if used well. We can not afford to not invest in it even if there are risks and likely several failures along the way. As Winston Churchill said, success is going from failure to failure without loss of enthusiasm.
Like what you read? Sign up for my newsletter at jan@janbosch.com or follow me onjanbosch.com/blog, LinkedIn (linkedin.com/in/janbosch), Medium or Twitter (@JanBosch).