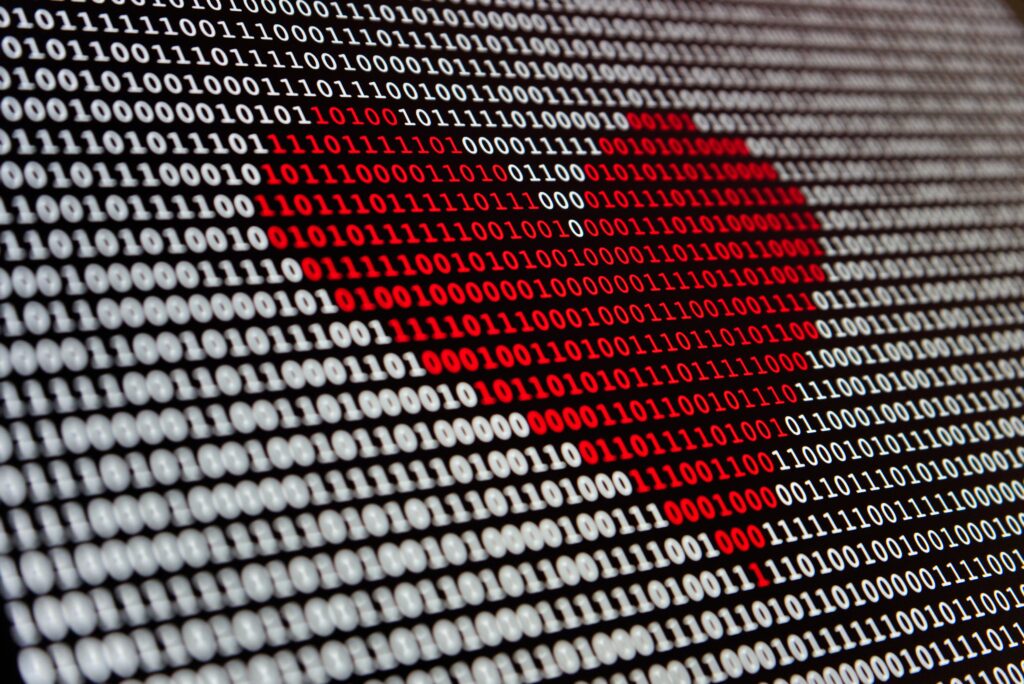
Imagine the following scenario. A (sizable) team at a large company writes customer documents in response to customer requests. They request help from the automation team to reduce their repetitive tasks. The automation team brings in an AI company, which develops an ML model that generates the customer documents automatically and virtually eliminates the need for human involvement. The prototype works amazingly well and both the AI company and the automation team are eager to move it into production as the cost savings, as well as the speed and quality of response to customers, are bound to improve significantly.
Sounds like a success story, right? Well, in this case, as well as in other cases that I’ve seen, the company managed to grab defeat from the jaws of victory. The solution wasn’t deployed. It’s probably not the end of the story and hopefully, the solution will be rolled out in the future, but the company experiences a significant delay in reaping the benefits from what should have been a straightforward and obvious deployment.
The pattern as I’ve seen it is that if AI is used to improve some product capability and it doesn’t affect existing organizational units nor existing processes, the deployment of the ML/DL model is quick and fairly seamless. The moment, however, existing organizations or teams are threatened in their existence or asked to reduce significantly in size or when existing work processes need to be adjusted to achieve the benefits, things rapidly grind to a halt and many in the organization start to backpedal.
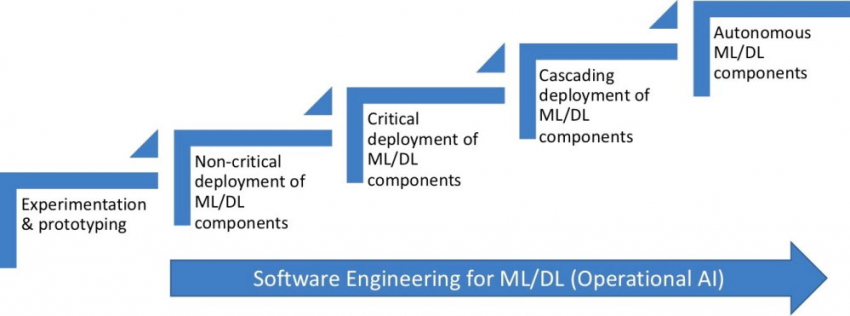
In an earlier post, I presented the stages that companies go through when adding ML/DL to products. As shown in the figure, the first stage is experimenting & prototyping. Every company I work with has a host of those initiatives ongoing. However, when looking to transition successful prototypes and proofs of concept to actual deployment, we run into roadblocks. advertorial
The first and obvious roadblock is that you now need AI engineering to ensure that you have industry-strength, production-quality deployment of AI and, as I discussed in an earlier post, that requires a set of solutions, architecture, infrastructure and processes that are often not recognized by data scientists and people without an engineering background.
The second and more important roadblock is that the potential of AI is to significantly reduce cost while improving speed and quality. The fact is that for most companies, the primary cost driver is salaries. So, to reap the benefits of AI, it means reallocating or releasing the people that currently are doing the job that will be replaced by ML/DL models.
This is so obvious that it’s almost painful to write it down and not feel like an idiot, but I keep running into situations like the scenario that we started with. Everyone loves AI and it’s on the top of the hype cycle. Everyone talks about all the great opportunities and benefits that AI will bring to their organization and society at large. But when it hits close to home, the willingness to change and reap the benefits suddenly is severely lacking.
This is a problem as the competition isn’t sitting still. We need to go through the painful process of reaping the benefits by reducing cost, redesigning processes, reallocating people and aligning your organization with the benefits that AI can offer. As I wrote earlier, it’s not what AI can do for you; the question is how you redesign your entire organization, business models, products, customer engagement models and ways of working to align with digitalization, meaning software, data and AI. This is the only way to capture the potential of AI to the full extent and the only way that you stay competitive in the long run.
In the startup community, large companies are often referred to as dinosaurs, ie slow, set in their ways and consequently ripe for disruption. Don’t be a dinosaur!
To get more insights earlier, sign up for my newsletter at jan@janbosch.com or follow me on janbosch.com/blog, LinkedIn (linkedin.com/in/janbosch) or Twitter (@JanBosch).