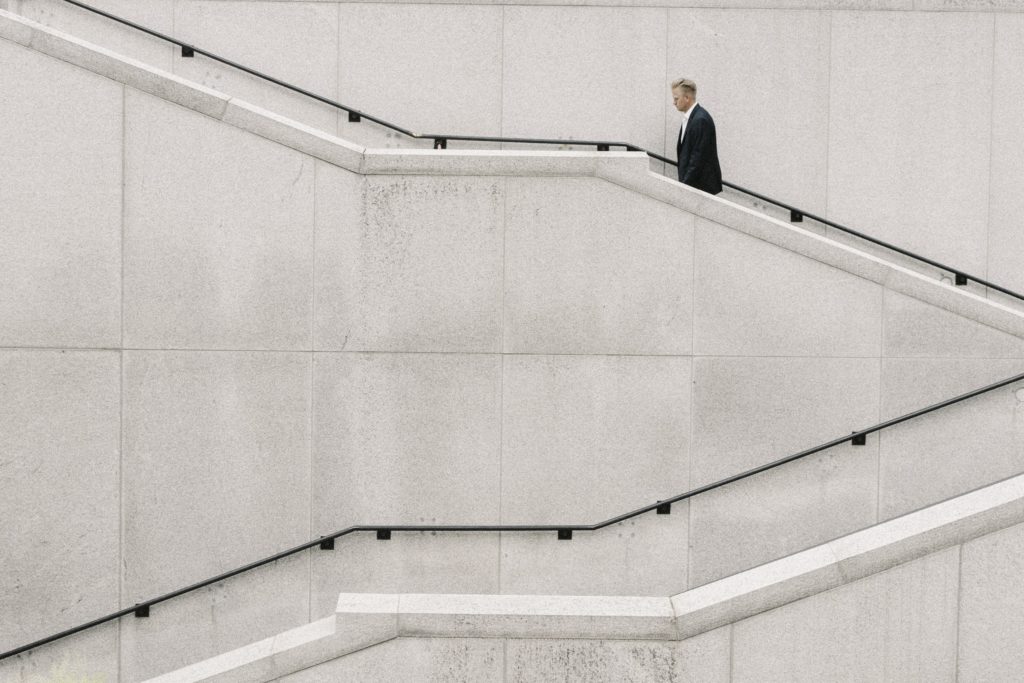
Having worked with companies on the use of AI, I’ve noticed an interesting pattern: although most of the attention is spent on algorithms, data storage infrastructure, training and evaluation of applications, the hardest part very often seems to be coming up with a promising concept in the first place. When exploring promising concepts, many start to realize that taking the resultant ML model from the prototyping phase to real deployment is a major challenge that requires changes in existing customer engagement models, product architectures, ways of working, the data collected and often even legal constraints.
Exploring promising concepts, of course, requires exploring both the potential business benefits and the expected cost for introducing a machine or deep-learning model in a product, solutions or service. However, my observation is that many struggle quite a bit with coming up with potential concepts that exploit the benefits that ML/DL models provide.
Earlier, we introduced the HoliDev model, which distinguishes between requirements-driven, outcome-driven and AI-driven development and claims that each type of development has its own characteristics. AI-driven development thrives where, on the one hand, there’s sufficient data available for training and operations and, on the other hand, we’re looking to solve an inference problem that’s particularly hard to solve without the use of ML/DL techniques as there’s no clear algorithmic approach. In general, we focus on three main characteristics that provide the key preconditions for a successful AI concept, ie removing hardcoded responses, using ignored data and revisiting negative RoI use cases.
First, in situations where the system response is hardcoded, there can be a significant benefit to providing a response to each request based on the available information. The obvious example is in the online advertising space where companies like Google and Facebook are constantly looking to create more accurate profiles of users in order to serve more relevant ads, rather than showing people a random ad. AI models can, especially when a good algorithmic approach is lacking, provide better responses by training based on available data.
Second, there are numerous situations where available data simply is ignored as humans haven’t been able to detect patterns in it and consequently follow a mathematical approach to solve a particular problem. An interesting example can be found in control systems where several companies are working to complement or replace traditional P, PI, PD and PID controllers with AI models. The reason being that traditional controllers operate based on a theoretical model of how a system is supposed to behave in response to control signals. In practice, no real-world system responds completely in accordance with the theory and AI models can improve the quality of control by taking all data into account.
Third, the most difficult case is where the cost of collecting data for human interpretation has had a negative return on investment as the effort required to benefit from the data was too high. With the decreasing cost of sensors, computing resources and communication, however, more and more cases exist where collecting the data for use by an AI model is actually becoming profitable.
It’s in this category where the most rewarding AI business cases can be found. One well-known example is sentiment analysis in social media. The amount of data in social media vastly outweighs the ability of even large teams of people to keep track of the sentiment around, for instance, a product or a company and consequently people didn’t even try. With the emergence of ML approaches, however, it becomes entirely feasible to have real-time dashboards of the state of the sentiment and companies use these insights for decision-making.
Concluding, for all the focus on AI algorithms, data and training, one of the most challenging activities remains the identification of interesting business cases and evaluating the feasibility and desirability of each case. I’ve discussed three categories of cases that can provide inspiration for identifying your AI business case.
To get more insights earlier, sign up for my newsletter at jan@janbosch.com or follow me on janbosch.com/blog, LinkedIn (linkedin.com/in/janbosch) or Twitter (@JanBosch).