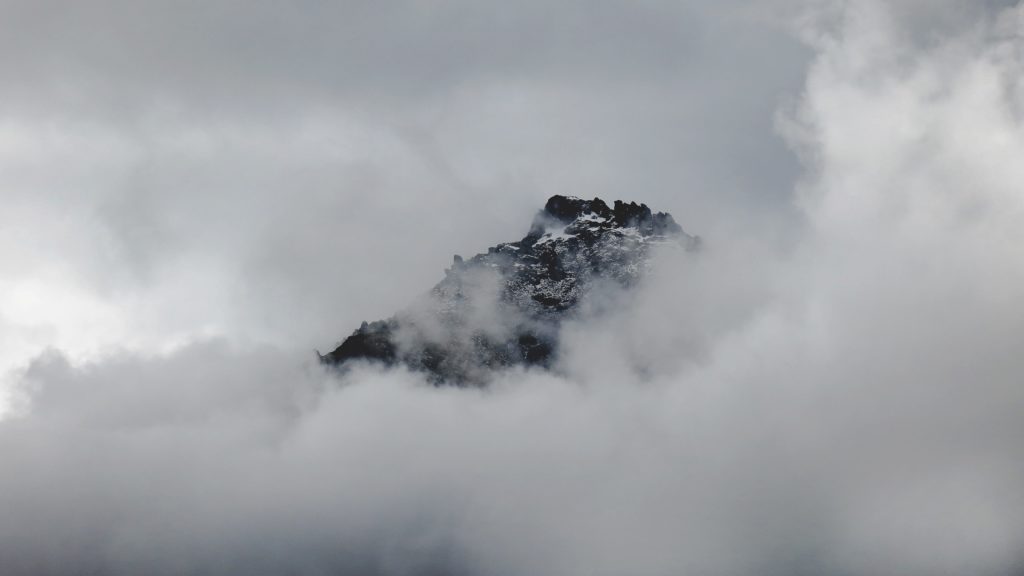
Last week, I wrote about the different types of use for data that we have available. That led to discussions with various people and I realized that there’s a problem around data that’s very typical in companies that have their roots in embedded systems or mechanical engineering: it’s actually unclear who owns the data from their products.
Most of the people in R&D that I’ve talked to over the last years couldn’t answer the question of who owns the data from their products. And those that did answer basically assumed the customer to be the owner. The first action that every company needs to take is to sort out the status around the data from a legal and practical perspective. It’s critically important to avoid a situation where this is unclear to customers and to your own staff. When it’s not clear, your staff will play it safe and avoid any use of data in order to avoid causing trouble.
Once you’ve sorted out the ownership and usage right issues, the next step is to figure out how data could be used for creating value for customers. The challenge is that even if you legally own the data, customers often still feel quite skeptical towards this and it’s important to be transparent about what data is collected and stored and for what purposes.
Using data to create value for customers often evolves through five stages. The first stage is broadly known and has been used for decades in most companies: quality assurance. Most customers have shared data from their products with the company developing the products in order to fix defects and to improve the general performance.
Once data collection for quality assurance is in place, the R&D organization can easily move to the next stage where R&D effectiveness data is collected. This can include the usage frequency of newly developed features, user experience data or other aspects that provide indications of business value delivered to customers.
The third stage is where you use the customer’s data to provide data-driven services based solely on that data. Examples of services may include general uptime metrics, preventive maintenance, reconfiguration times in manufacturing lines and general productivity metrics. The advantage of these data-driven services is that they can run on the customer’s premises without any concern. One question in this context is why the customer would need the company to provide these services as they already have the data themselves. In practice, however, interpreting the data requires a deep understanding of the system, which the customer often doesn’t have.
Once customers are used to the company using their data to deliver services, the next stage is to offer them data-driven services that combine their data with anonymized, aggregated data from the entire customer base. This is where potential sensitivities concerning competitive positioning start to play a role and where the company has to provide guarantees concerning anonymity and confidentiality. In most cases, the starting point is where a number (typically five or more) of the largest customers jointly agree to their data being used for this purpose. Many companies use the data available in this stage to offer consultancy services to help their customers improve their performance.
In the fifth stage, the company offers data-driven services based on data originating from other sources besides its primary customer base. This can include data concerning the customers of their customers, data from adjacent industries that pertains to this industry in different ways or general data concerning the economy, currencies, weather, politics or other topics that can have a material effect on your customers.
Concluding, if it isn’t clear already, the first step has to be to create clarity and transparency concerning the ownership and usage rights of data coming from your systems deployed at customers. Typically, this requires giving customers something of value that will make it relevant for them to share their data. The use of data often evolves through five stages, as outlined. But start with getting your data out of the gray zone.
To get more insights earlier, sign up for my newsletter atjan@janbosch.com or follow me on janbosch.com/blog, LinkedIn (linkedin.com/in/janbosch) or Twitter (@JanBosch).