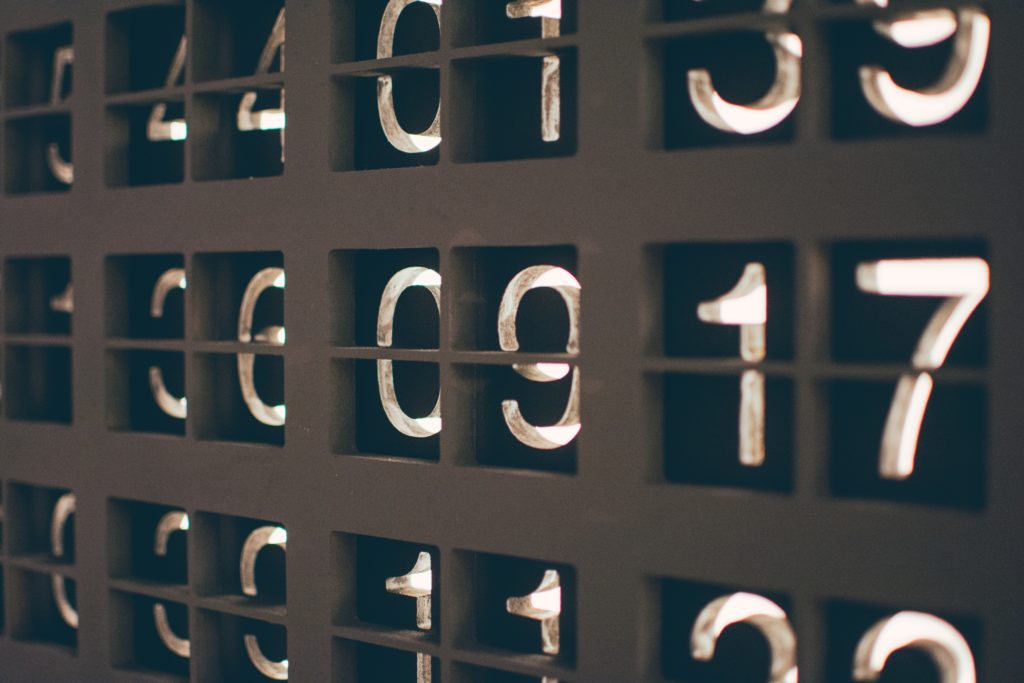
If you’ve been reading my posts, you know that I feel data is one of the key ingredients of a successful digital transformation. It’s not just about adding software to your products or putting DevOps in place. It is as much about collecting, analyzing and storing data and using this data to improve a variety of aspects of the business. As, in my experience, every proponent of the use of data has a specific and different interest from others, the interesting question is what these relevant aspects are. Here, I provide an initial taxonomy of the uses of data.
At a top level, we can distinguish between the use of data inside and outside the company. Internal use can often be broken down into the use inside the R&D organization and the use in the rest of the company. Inside the R&D organization, data has traditionally been used for quality assurance of products out in the field. During recent years, at least three purposes have been added to that: analytics, experimentation and artificial intelligence/machine learning/deep learning.
Inside R&D
Analytics
The first is the use of data for analytics purposes. This includes gaining an understanding of the number of users, the frequency of use of different features and user experience issues such as aborted actions. In this case, data is used to determine whether our model of the customer and the system in the field is aligned with reality.
Experimentation
The second use of data inside the R&D organization is concerned with experimentation. In those areas where the analytics show that our understanding of the customer or the deployed system is lacking, teams can use experimentation, such as A/B testing, to try out in the field what the best way for realizing features and system functions is. The best way to perform this from a statistical perspective is to run fully randomized experiments, but in practice, also less rigid experimentation can generate valuable insights.
AI/ML/DL
The obvious use of data that has gained enormous popularity in recent years is the use for training machine learning and deep learning models. Due to the availability of data and significant improvements in the performance of hardware architectures such as GPUs, DSPs and ASICs, the use of artificial intelligence has become a highly beneficial option for many application domains. However, ML/DL models are very data hungry and often need large amounts of (labeled) data for training.
Outside R&D
Moving to the use of data inside the company, outside of R&D, the primary focus is often on the financial impact of decisions – in the entire organization. Typical goals include value modeling, tracking performance of teams and, again, experimentation and AI/ML/DL.
Value modeling
One of the key challenges in companies is to align activities at all levels in the organization. One of the most effective approaches is to build a hierarchical value model where the top-level business KPIs are translated into lower-level metrics with quantitatively defined relationships between them. This value model then uses any data from the field or from customers to infer other factors.
Tracking performance of teams
Many companies also use data to track team performance in sales, customer support and other functions. Although this isn’t a new concept, digitalization allows for the use of much more data, as well as different types of data. This gives more precise and detailed insight into teams.
Experimentation
Most people assume that A/B testing was invented by the SaaS companies, while in fact, it’s a technique originating from marketing in the mid-20th century. So, data-driven experimentation can be used across the company and many digital companies do apply these principles also outside of R&D.
AI/ML/DL
Similar to experimentation, the use of ML/DL models is applicable outside the realm of products as well. For example, in customer support, such models can be used to more rapidly help a customer resolve a problem by classifying symptoms into likely issues and, of course, to recommend the most likely cross- and up-sell suggestions.
Outside the company
Shifting perspective from inside to outside the company, the use of data can be separated into two main areas: providing data-driven solutions to your existing business ecosystem, such as your customer base, suppliers and partners, and monetizing data from your primary customer base with a secondary customer base. For the former, some typical uses of data include preventive maintenance services to customers, business performance analytics and alternative business models.
Preventive maintenance services to customers
The most obvious case that has received lots of attention in the IoT communities is the notion of preventive maintenance. By measuring systems during operation and having data from many system instances, the company can detect likely component failures before they happen and then recommend maintenance at planned downtime periods rather than suffer from breakdowns.
Business performance analytics
As the company has multiple customers and it receives data from all of these, it can provide a service that allows each customer to compare its performance to aggregated and anonymized data from others like it. This even makes it possible to offer consultancy services to help customers improve their business performance.
Alternative business models
As the company can now measure the value it delivers to customers, it can use alternative business models based on value-based pricing. This often includes forms of continuous improvement where the company delivers solutions that improve the customer’s business performance and is reimbursed a part of the value created for the customer.
The final category is concerned with monetizing data from the primary customer base with a second customer base. The mechanism of a two-sided market is difficult to put in place but the benefits are enormous as it often allows the company to use the funds from its second customer base to subsidize its primary customer base and through that, increase its market share. There are two broad categories of monetization with secondary customer bases: aggregate activity data and customer profiles.
Aggregate activity data
The activity from the entire customer base or a part of it can be aggregated and offered to others that can use this data to improve their value offering to their customers. As a hypothetical example, a company selling connected trucks could calculate the total kilometers driven in aggregate across the entire customer base every week in every country in Europe and offer this information to companies that sell economic activity data. As the amount of goods transported is a good indicator of the amount of economic activity in a country, this could be valuable for previously unrelated companies.
Customer profiles
Rather than selling aggregate data, the company can sell anonymized (or not) customer profiles that others can use. For instance, many online businesses sell your profile data to ad networks, allowing these to serve more relevant ads to you and through that, improve their effectiveness. This category often is criticized by many as they feel that their data is used unfairly. However, it has to be obvious that if you get to use an online service for free, the data trail you generate is used to generate revenue from other parties.
Concluding, many agree that collecting and using data is an integral part of a digitalization strategy. The challenge is that they have one specific use in mind and don’t think in terms of a holistic view of the types of data and the possible uses of that data. My goal was to outline a first, high-level taxonomy of the use of data. What data does your company generate and collect and how can you use this data to the advantage of you and your customers? What use is your data?
To get more insights earlier, sign up for my newsletter atjan@janbosch.com or follow me on janbosch.com/blog, LinkedIn (linkedin.com/in/janbosch) or Twitter (@JanBosch).