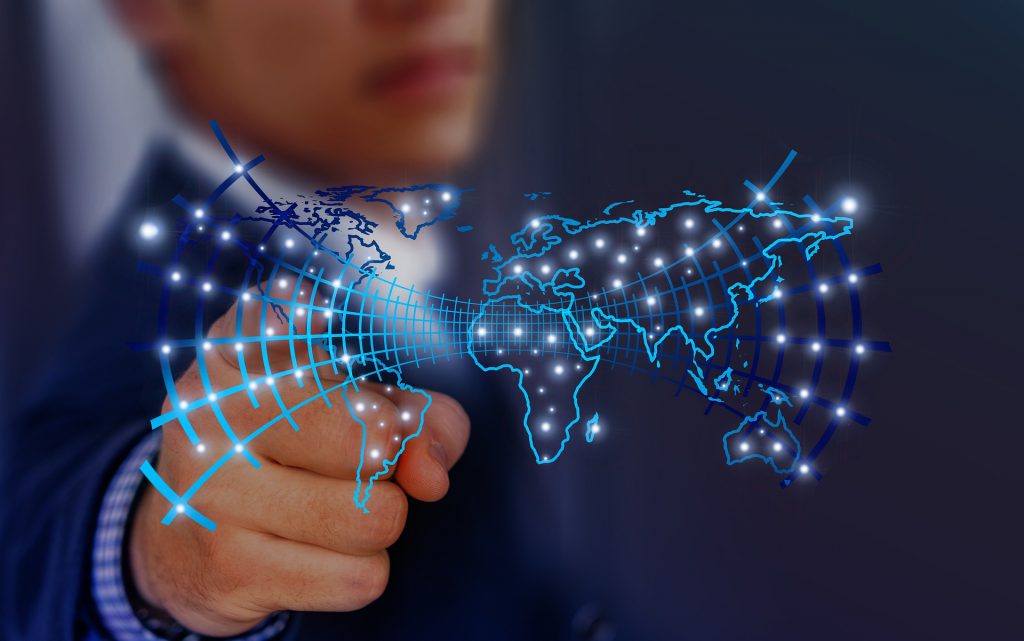
During the summer, I spent a week at a summer school on deep learning (DL). There were several reasons to attend, but one was to simply learn more about this trending topic. In many ways, it was a wonderful, though humbling, experience as the field is progressing at a rate that’s simply phenomenal. There are constant breakthroughs in the area and the quality of models, in terms of accuracy and loss, is still improving rapidly with every year significant improvements being reported by various research groups (especially the industrial ones).
The ability of the prototypes presented during the sessions was amazing. For instance, machine learning (ML) now exceeds the ability of theoretical physicists to classify data in high energy physics. Deep learning models generate images that are amazingly realistic to the point of being indistinguishable from real photos. Classification in a wide range of problem areas is exceeding human ability, such as in medical images and understanding human speech.
Although machine learning traditionally was applied on data that initially was intended for human consumption, researchers and engineers are starting to realize that data at that level already has been processed and abstracted quite significantly. Consequently, there’s a tendency to move machine learning closer to the lower levels in systems where the raw data is generated. This allows ML techniques to detect features in the data that have been filtered out of higher-level data that has been processed for human consumption.
The consequence is, of course, that we’re entering an interesting paradox. On the one hand, ML techniques are implemented closer to the places where raw data is generated – typically, sensors and devices that are deployed in the real world. These systems often have quite limited computational resources. On the other hand, the closer we are to the raw data, the larger the quantity of data actually is in practice. This requires careful balancing of computational resources and generated value – a trend that will fuel the continuous development of more powerful and more energy-efficient computation.
Nevertheless, the trend is clear: AI and specifically ML and DL will be embedded in the fabric of computing. Everywhere where data is generated or processed, ML/DL algorithms will evaluate whether there are ways to improve the outcome of system operation by classification, prediction and generation.
Of course, today, we’re far from this reality but during this summer school, it became clear to me that the world clearly is moving in this direction. The challenge is what companies will control this new technology space. In some areas, such as natural language processing, the most advanced algorithms require such enormous amounts of computational resources that only the FANGs of this world, ie companies like Facebook, Apple, Amazon, Netflix and Google, will be able to use the techniques. Similarly, it’s clear that in machine learning, larger data sets almost always lead to superior outcomes. In one paper that was discussed during the summer school, the worst algorithm with ten times the data performed better than the best algorithm with one time the data. This means that there’s a positive feedback cycle between the size of the company in terms of customers and the quality of solutions it’s able to put on the market as it’s typically the customers that generate the data that’s used for training purposes.
Although I’m a happy customer of most of the FANGs, I do think that especially when it comes to AI/ML/DL, we should aim for democratization where companies of all sizes, as well as individuals, enjoy a level playing field – hence my involvement with Peltarion. Embedded systems companies need to have an explicit strategy to control their data, hypothesize the most promising use cases, experiment with different ML/DL algorithms and find suitable ways to serve their customers better through data and AI.
It sounds like a platitude but the fact is that data is the new oil. Software in general and ML/DL in particular constitute the technology that refines the data and generates real, tangible value from this data. We can’t afford to only have the FANGs of this world exploit this capability and I’m glad that companies such as Peltarion are looking to democratize the use of AI. However, it doesn’t free you from the obligation to figure out how your company will be using data and AI to the advantage of your customers.
To get more insights earlier, sign up for my newsletter atjan@janbosch.com or follow me on janbosch.com/blog, LinkedIn (linkedin.com/in/janbosch) or Twitter (@JanBosch).