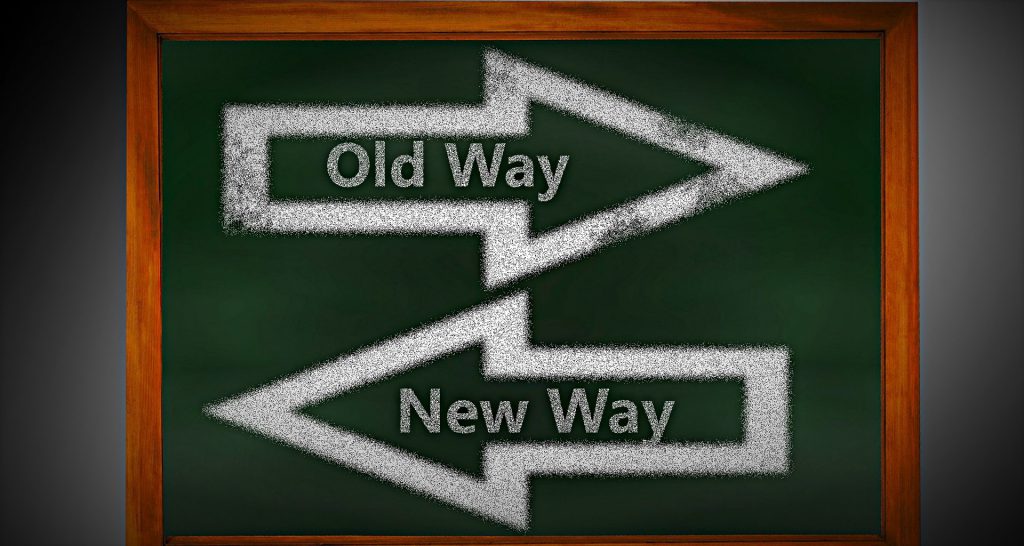
Both the proponents and questioning antagonists around artificial intelligence are expounding on the outrageous capabilities of modern AI solutions such as LLMs, foundation models and agentic AI. And yet, when interviewing key business leaders and observing the companies I work with, I must say that the adoption of AI is slow going and far from the enormous upheaval many claim it will result in.
So, what’s going on here? On the one hand, AI is presented as either our greatest nemesis that will kill us all or the second coming of Christ that will bring us all to Nirvana. On the other hand, for all the noise around AI, its adoption in industry is slow-moving and not as disruptive as what many had feared or hoped for. At least until now, as many would say …
One aspect that many fail to incorporate in their predictions is the notion of innovation absorptive capacity. Humans, companies, industry ecosystems and society at large can absorb new technologies and other innovations at a limited pace. One illustrative example is the introduction of electric motors in manufacturing companies in the late 19th century. Before this, there was a central steam engine in the factory and the rotational energy was distributed via axles and bands. Initially, companies simply replaced the steam engine with one huge electric motor. Close to three decades went by before all manufacturing had adopted small electric motors in each machine in the factory rather than a centralized one. Even if the safety and efficiency improvements were obvious to everyone, the broad adoption of the new technology still took decades.
Since then, we’ve seen an ever faster adoption of new technologies as they’re introduced to society. For example, ChatGPT reached 50 million users in just two months, whereas earlier offerings such as Instagram, Facebook and Twitter needed more than a year or several years. However, when it comes to changing the ways of working in companies and automating process steps or entire processes that weren’t previously feasible to be automated, things go much slower. Many people are using tools such as ChatGPT as personal productivity improvement tools or simply out of curiosity – the stage we refer to as “playtime” – but changing beyond that requires coordination and alignment with many in the organization, as well as existing ways of working, which complicates adoption.
Several challenges are slowing down the adoption of AI in companies. These include identifying clear use cases, organizing the data necessary for agents, agreeing on clear, quantitative outcomes, establishing the suitable level of human oversight and control, integrating agents in existing workflows, ensuring compliance with regulation, technical challenges and, not least, organizational and cultural resistance in the organization. I’ll discuss each of these in more detail.
First, one of the patterns I’ve identified in the conversations I’ve had around the adoption of AI is that people find it much easier to identify opportunities in other people’s jobs than in their own. I hypothesize that they only see the superficial, obvious aspects in other jobs but are intimately aware of the complexities and nuances in their own work. So, identifying use cases is proving surprisingly difficult in many companies.
In this context, one complicating factor is that many companies haven’t defined clear success metrics or KPIs for tasks and processes. When the quantitative outcomes aren’t well defined, it’s often hard to determine whether an AI-based solution is performing at an acceptable level or not. In customer support, for instance, human agents also make mistakes when interacting with customers, but the rate of failure is often not measured or tracked. When changing from human customer support agents to AI agents, we now lack a clear baseline for what constitutes acceptable performance.
Often, the notion of trust comes up: how do we know we can trust the AI agent? The implicit but obviously incorrect assumption is that humans are 100 percent trustworthy and never make mistakes. However, as we don’t measure their performance, we have no metric to compare an AI agent with. As that agent will make mistakes too, the general tendency is that the AI-enabled solution is inferior and can’t be trusted.
A second challenge is data. For any AI solution to work properly, the data given to it for inference or training needs to be of sufficient quality. The adage of garbage in, garbage out is as true for AI as it is for any other situation. The challenge is that many companies may treat their software with a certain level of professionalism, including proper testing, versioning and certification, but their data is often managed at not nearly the same level. Individual teams can start and stop data collection at will, services can be based on raw data without proper cleaning and semantic definition and, especially in software-intensive systems companies, the data is often used for quality assurance and diagnostics and lacks contextual data necessary for training ML models. Adopting AI models or agents requires companies to embark on a journey to mature their data practices, which is often challenging as it requires many decisions on what to collect, at what frequency, where to store it, how to process it and how to make sure it can be used both for training and inference.
A third challenge is concerned with cultural and organizational resistance, as well as integrating AI solutions into existing workflows. To reap the benefits of AI, companies need to redesign their business processes in an AI-first fashion, meaning that we start from scratch, determine what can be performed by AI agents or ML models and only then identify specific tasks or process steps to be conducted by humans. When done properly, this typically results in a fundamental overhaul of current ways of working, which tends to result in significant resistance from people. Both because change in general leads to resistance, but even more so because it tends to result in sizable efficiency improvements, meaning that the same work can be done by fewer people, causing people to worry about their jobs.
One topic that often comes up is that the AI-enabled solution needs to be as good as or better than a human-driven solution. That’s obviously not the case. The solution simply needs to have better economics. As an example, in modern supermarkets, self-checkout is increasingly becoming the norm. The store owners know that this leads to higher levels of theft, as well as items that were accidentally not scanned to be taken home. However, the higher losses are, by far, outweighed by the reduced personnel cost for cashiers. It simply is a more economical solution. The same is the case for AI-enabled solutions.
The fourth and final main area is concerned with regulatory compliance and human oversight. For all the attempts to be concrete and specific in regulations, there’s frequently a level of human interpretation required to ensure compliance. When applying AI solutions in areas where regulatory compliance is a concern, both regulators and, where applicable, certification agencies, are often unclear on how to achieve compliance using agents. This uncertainty causes companies to avoid or slow down AI adoption, as it’s unclear how to deploy the solutions. Without deployment, the benefits of the solution won’t be achieved, so there’s no point.
For all the promise of machine learning and agentic AI, we see that the adoption beyond the individual in most companies is still quite limited. Beyond the generally low innovation absorptive capacity of companies and industries, there are several challenges to be considered. These include finding suitable use cases, ensuring sufficient data quality for both training and inference, organizational resistance and regulatory compliance concerns. To end with a quote from Tim Cook: “What all of us have to do is to make sure that we’re using AI to the benefit of humanity, not to the detriment of humanity.”
Want to read more like this? Sign up for my newsletter at jan@janbosch.com or follow me on janbosch.com/blog, LinkedIn (linkedin.com/in/janbosch) or X (@JanBosch).