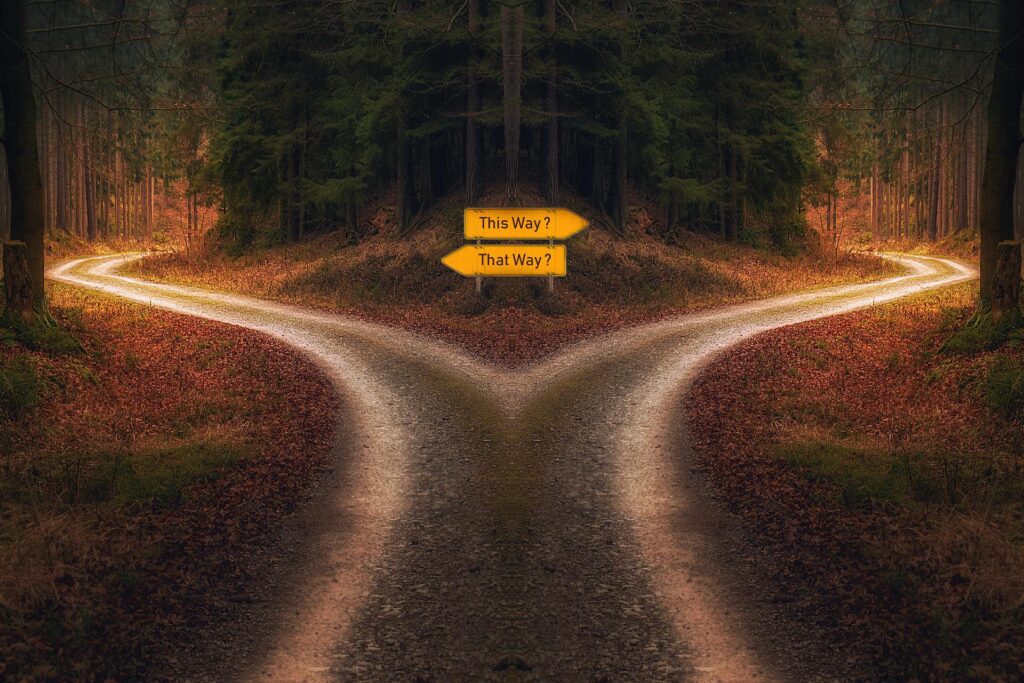
Although it is uncertain who said this, a famous quote is “It ain’t what you don’t know that gets you into trouble. It’s what you know for sure that just ain’t so.” This notion is at the heart of digitalization: much of what we think we know is no longer true when the industry goes through a digital transformation.
Successful companies typically know something that the rest of the industry does not and this generates their success. This “secret sauce” may benefit the company for a long time, decades even, but when significant disruptions happen, all assumptions need to be revisited and reevaluated.
Most companies hold what we refer to as shadow beliefs. These are beliefs that may have been true in the past, but that are no longer true today. However, all in the company still operate based on the shadow beliefs and, in a sense, are focusing their energy on the wrong thing.
The only way out of this conundrum is to continuously test your assumptions with customers and the market and one of the most effective ways to do this when it comes to the product is by conducting experiments. When designed correctly, experiments provide statistically validated results that provide irrefutable evidence concerning the hypothesis that underlies the experiment.
In software intensive systems, experimentation is often conducted in the form of A/B tests. The basics of an A/B test are exactly what the name implies: we develop an A alternative and a B alternative for a particular feature or aspect of our offering. We deploy both the A and B alternatives and randomly assign users to the A and B groups. We measure the behavior of the A cohort and the B cohort and once we have enough data to derive a statistically validated conclusion, we deploy either the A or the B alternative to everyone and conclude the experiment.
When working with traditional companies on introducing A/B testing, one of the interesting topics that often comes up is that it is actually unclear to most in the company what they are optimizing for. For instance, in a workshop with a company in the automotive domain, we discussed adaptive cruise control (details are deliberately vague). However, when trying to quantify what constitutes a better adaptive cruise control feature, it became clear that the dozen or so people in the room did not have a clear view on nor alignment on what a successful adaptive cruise control looks like.
The consequence of vagueness on the desired outcomes is obvious: inefficiency. When different people have different views on success, they will each take decisions and act in a way that aligns with their own beliefs. And these decisions and actions may easily conflict with each other, potentially canceling each other out. Secondly, even if you align within the company on what constitutes success, it may still not be what the majority of customers would prefer.
Digitally born SaaS companies tend to run thousands upon thousands of A/B tests continuously and as a result are extremely data driven. This requires these companies to be very clear on the factors that they are optimizing for, often resulting in a hierarchical value model (see also this post).
As we’ll discuss later in this series, we need the value model not just for A/B testing, but also for using AI models. Any model that is to be trained needs to know what is better and what is worse in order to optimize itself. You can do this through examples (labeled data sets), but also through quantitative models.
Concluding, traditional companies often suffer from shadow beliefs once they enter a digital transformation: the things that perhaps once were true are no longer true, but we act as if they were. In response, we need to become very precise and quantitative in terms of what we aim to optimize for and then validate any potential improvement using experimental techniques such as A/B testing. As the Cheshire cat told Alice in Wonderland, if you don’t know where you want to end up, any road will do. And most of these roads do not lead to where you want to go.
Like what you read? Sign up for my newsletter at jan@janbosch.com or follow me onjanbosch.com/blog, LinkedIn (linkedin.com/in/janbosch), Medium or Twitter (@JanBosch).