Go Radical: Double R&D Effectiveness
Over the last 30+ years, I have worked with a variety of companies, ranging from large, international companies to small startups and building anything from safety-critical embedded systems to mobile apps. Especially during the last 15 years, one of my main areas of interest and focus has been R&D effectiveness, i.e. how to ensure that every € invested in R&D leads to the highest return on investment.
The problem is that our research (as well as work by others) shows that the R&D effectiveness in many companies leaves a lot to be desired:
- Most companies spend 70%-90% of their R&D resources on commodity, non-differentiating functionality.
- For new functionality that gets built, at least half of that is used so seldomly, if at all, that it should not have been build in the first place.
One of the key root causes for this is that many companies are unclear what their customers consider to be valuable and what is considered to be commodity. Especially when asked to describe the value that is delivered to customers in quantitative terms.
We are convinced that companies are able to DOUBLE their R&D effectiveness, that is generate TWICE AS MUCH revenue from the same R&D investment, by changing the way the company conducts R&D, managed its products as well as its customer relations.
To achieve this, however, we can’t make small changes to the way that we do things today. Instead, we have to go RADICAL and adopt a different paradigm. The paradigm is organized around three principles:
- Get clear on what is the real value delivery to customers and capture this in a quantitative value model that can be tracked in real-time.
- Decrease investment in commodity functionality with at least 50%.
- Adopt an iterative, experimental approach to developing new functionality where the relevance of the new functionality for customers is continuously established in quantitative terms.
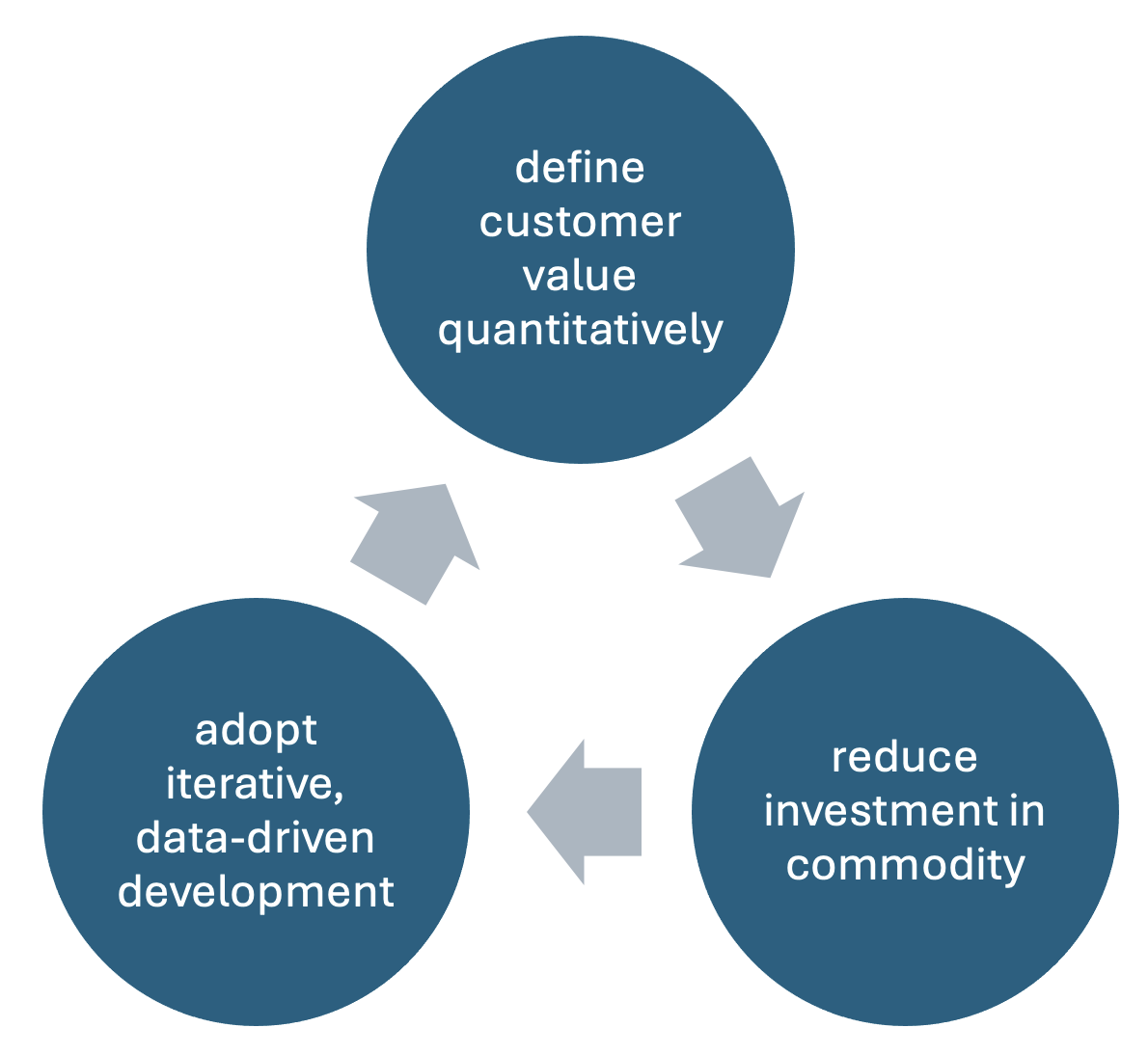
Over the last two decades, we have developed a set of tools that allow companies to achieve this outcome. These tools include:
- Hierarchical value modeling. See here for an introduction into the concept.
- Three layer product model to minimize investment in commodity functionality. See here for a high level overview or read this short book.
- Iterative and data-driven development of new features such as the HYPEX model. This short book gives an introduction into the topic.
- Tooling: we have started development of a tool that allows companies to track value delivery to customers in real time. We’d love to discuss this with you in more detail!
We are currently looking for companies are ready to Go Radical! Reach out at jan@janbosch.com and lets start on DOUBLING your R&D effectiveness!
If you are interested in the work leading up to us Going Radical, below you’ll find some more details on my work on digital transformation, platforms, artificial intelligence and data.
Digital Transformation
Digital transformation involves the transition from a transactional business model to a continuous business model where we continuously deliver value to customers. Typically, this requires techniques such as continuous deployment (or DevOps), value design, A/B testing, etc. However, the start is a fundamental shift of the interface to the customer as well as of the business model. There are many aspects to digital transformation and many organizations fail to address all relevant dimensions and fail to reap the benefits. Also, there is no recipe that works for everyone and each organization has to develop its own strategy and path. However, that does not mean that you have to go it alone. I can help!
To read more, here are some of my blog posts that might be interesting:
Or if you prefer to watch, here is a related talk on YouTube
Platforms and ecosystems
Few topics lead to as much discussion in companies as platforms. Having worked with platforms for most of the last 30 years, I have seen the notion of platforms evolve over time and today we can distinguish at least three main goals that companies have for platforms, i.e. platforms for reuse, platforms for DevOps and platforms for an ecosystem. Companies are often confused and unclear about their platform strategy, which leads to major inefficiencies and tension. I have worked with a variety of companies on their platform strategy, the link to the business strategy and the role of the ecosystem surrounding the platform.
To read more, this might be an interesting starting point: 10 learnings about platforms.
On YouTube there is an interview by Software Engineering Radio with me on the topic of platforms.
Artificial Intelligence
As one of the key digital technologies, no company can afford to ignore this transformational technology and every company that I work with has one or more prototypes and experiments ongoing. However, taking these prototypes and deploying these in real-world systems proves to be very challenging and many fail in this step. In response, we have worked hard on AI Engineering as a discipline to ensure that all the engineering required to successfully deploy machine- and deep-learning models in production are available. I work with several companies on addressing this challenge.
If you like to read more, this might be an interesting article: Towards autonomously improving systems
On YouTube you can watch me here:
Working with Data
Digitalization is concerned with three technologies, i.e. software, data and artificial intelligence and we need to address all three to successfully transition to becoming a digital company. For data, this means organizing the legal collection of data, data pipelines, data lakes, analytics, etc. However, data is also a key enabler for experimental development approaches such as A/B testing as well as for data-driven decision making and value design.
Some relevant reads include:
If you prefer to watch a talk: